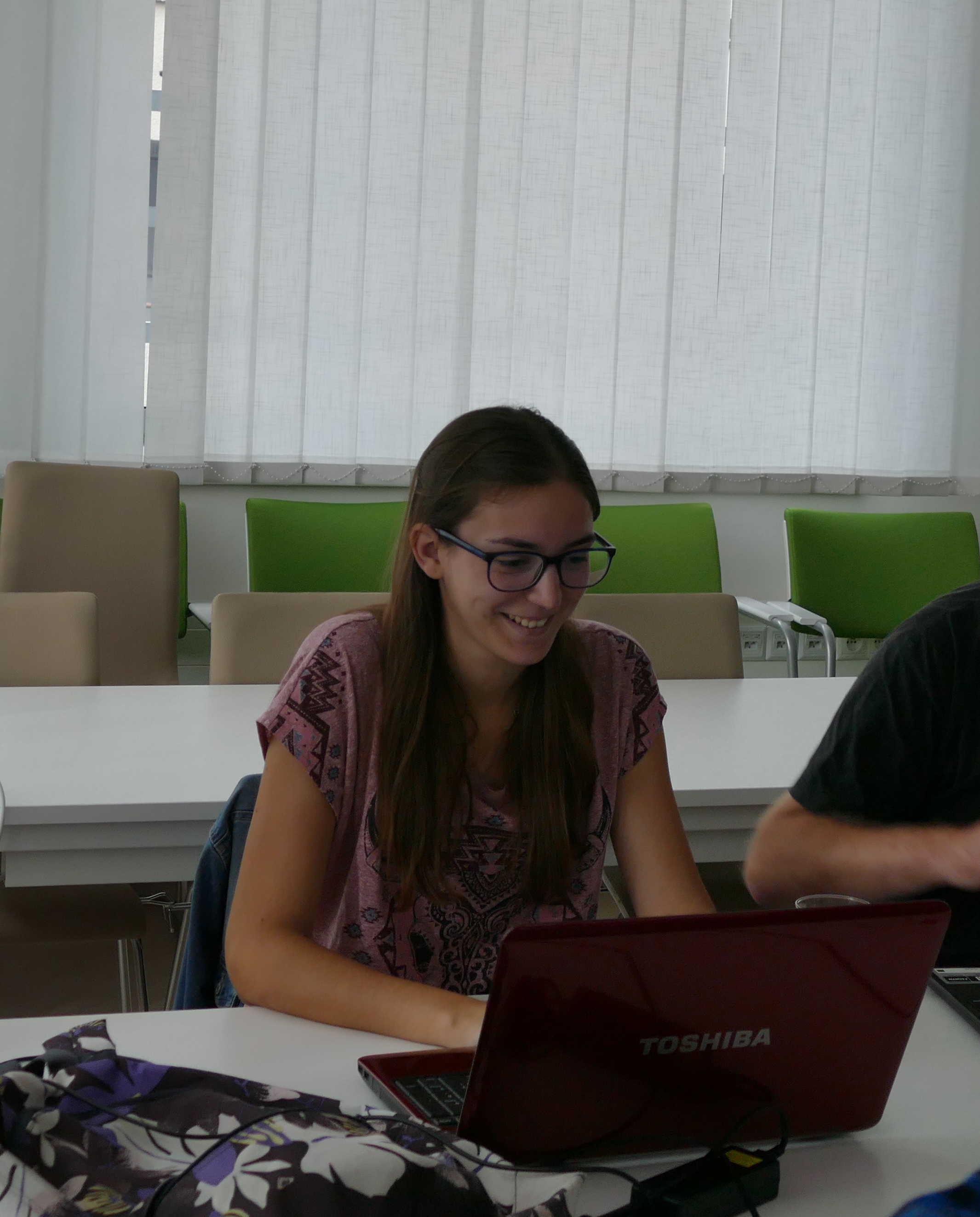
Hello world!
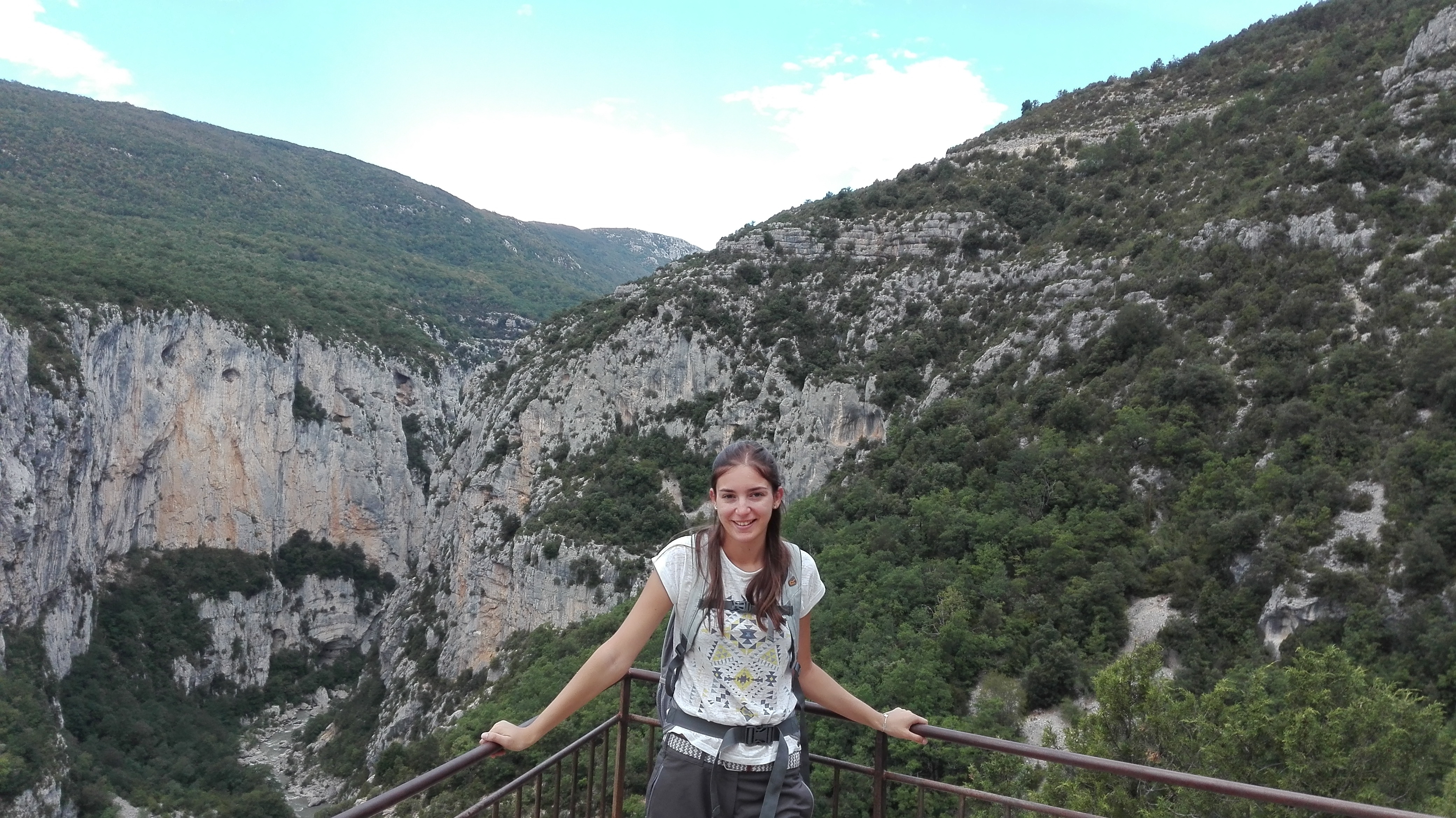
Happily hiking in France, minutes before getting lost in the Mountain.
My name is Ana María Montero and I am a 23 years old Physics student. I am from Spain and I come from a nice town close to the border with Portugal called Badajoz, where I also study. I am about to finish my Bachelor’s studies in Physics at the University of Extremadura where I am preparing my thesis regarding the behavior of classical one-dimensional liquids (which means I put a lot of marbles in a line and check their properties).
Next year I will be travelling to Germany to pursue my Master’s degree in Simulation Sciences offered by RWTH Aachen and Forschungszentrum Julich as I would like to specialize in computer simulations. This is the main reason why I applied several months ago to be here today, participating in the Summer of HPC program and working in the best computing centers in Europe.
Apart from this, and though it seems like debugging stuff is my favorite activity (if it is not my favorite one, it definitely is the one I dedicate most time to) I also enjoy doing a lot of other activities: Regarding the musical world, I play the piano and the violin whenever I can and I also sing in my university choir. Regarding outdoors activities, I love hiking (even when I get lost in the middle of a mountain in France) and traveling (even when that means that I have to face the heartless Polish winter).
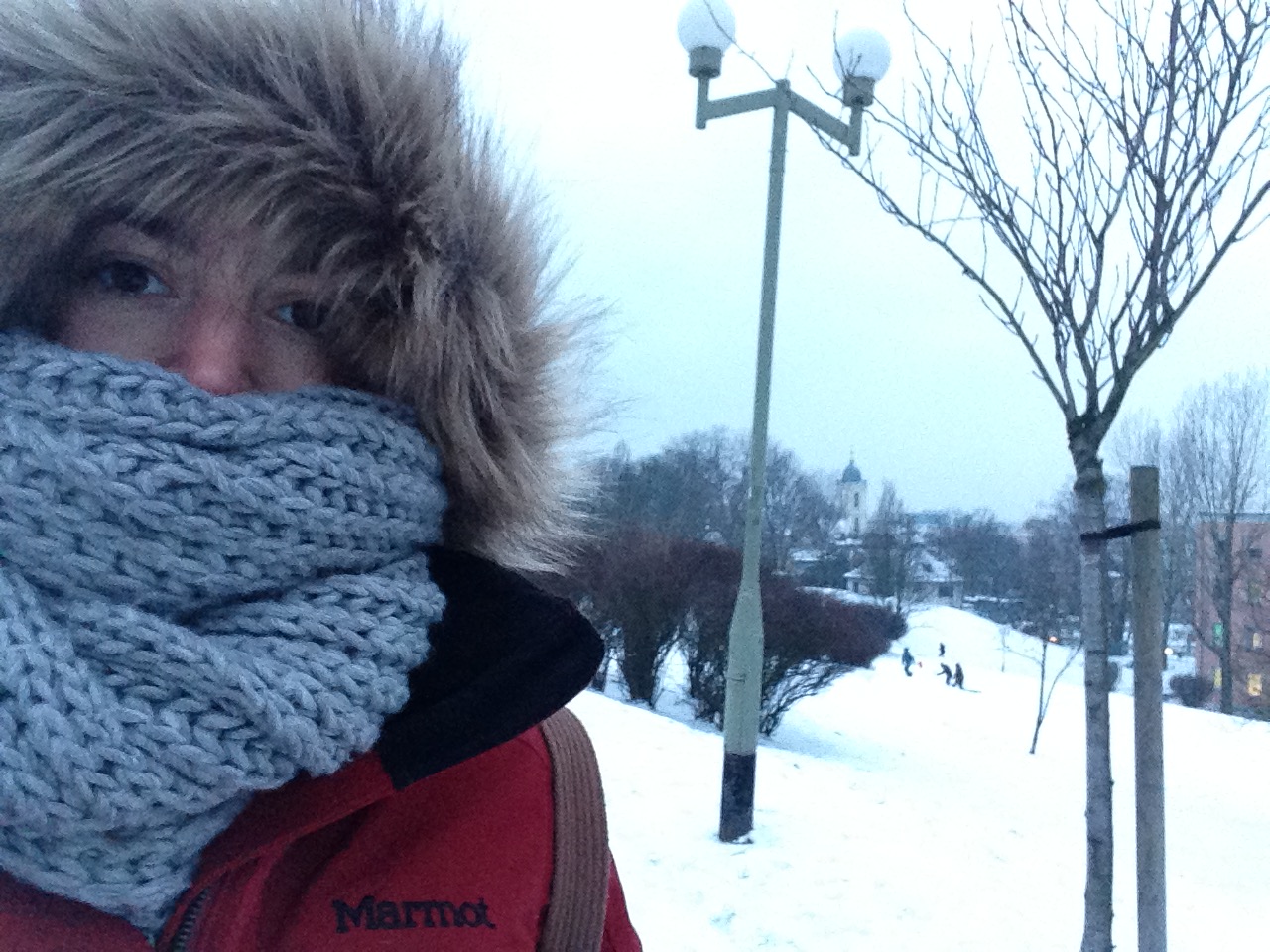
This is how a Spanish girl looks like in Poland in January.
This year’s vacation however, consists of spending a couple of months in Dublin, the city of Guinness, live music and Temple bar. But Dublin is also the city of ICHEC, where I will be working for two months studying and predicting “El Niño” and its consequences worldwide (who knows, maybe this helps Irish people predict their unpredictable weather). So, in general terms, I have already set up the algorithm for my summer plans, and, speaking in FORTRAN language, it looks like this:
—————————————————–
! Ana’s summer program 2017 for FORTRAN speakers: Do day=1st of July, 8th of July, 1 Amazing training week in Ostrava End do Do day=9th of July, 31st of August, 1 If (day==weekday) then Work at the ICHEC in Dublin Else Visit and enjoy Ireland! End if End do -----------------------------------------------------
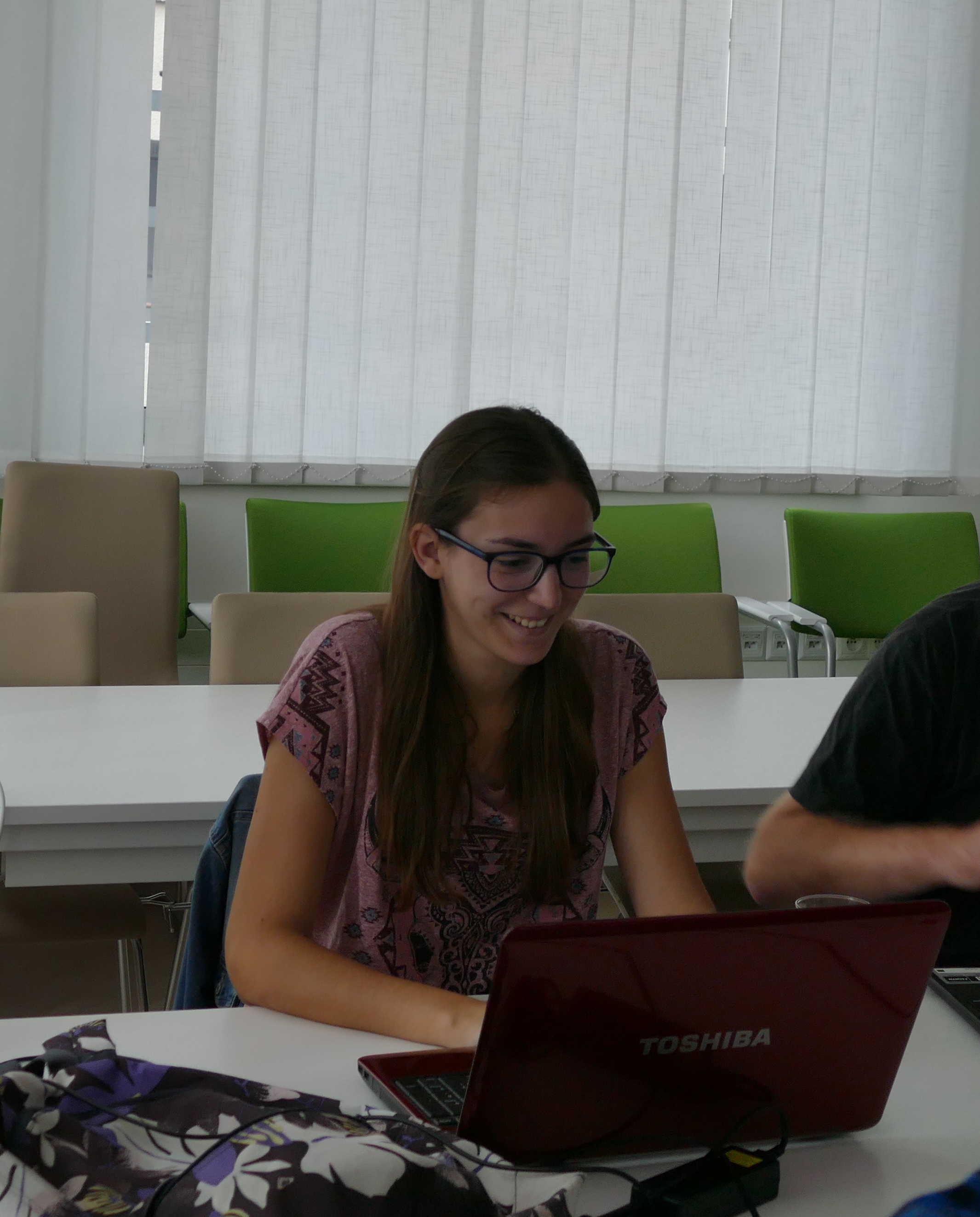
Enjoying the training week in Ostrava
Nevertheless, as amazing as the plans look like right now, by the end of the summer I will be able to show those plans to the world. My visualization work at the ICHEC will help me bring “El Niño” and its consequences to life and my camera will help me capture the rest of Ireland’s wonders.
After an incredible week at IT4Innovations in Ostrava, I cannot wait to start my project in Dublin and to put into practice everything I have learnt.