Selecting the true magical bullets
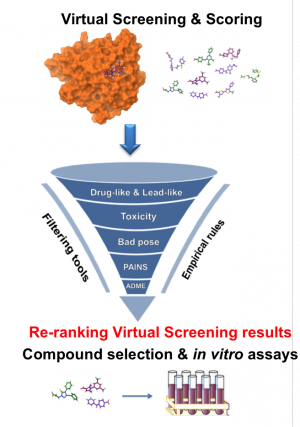
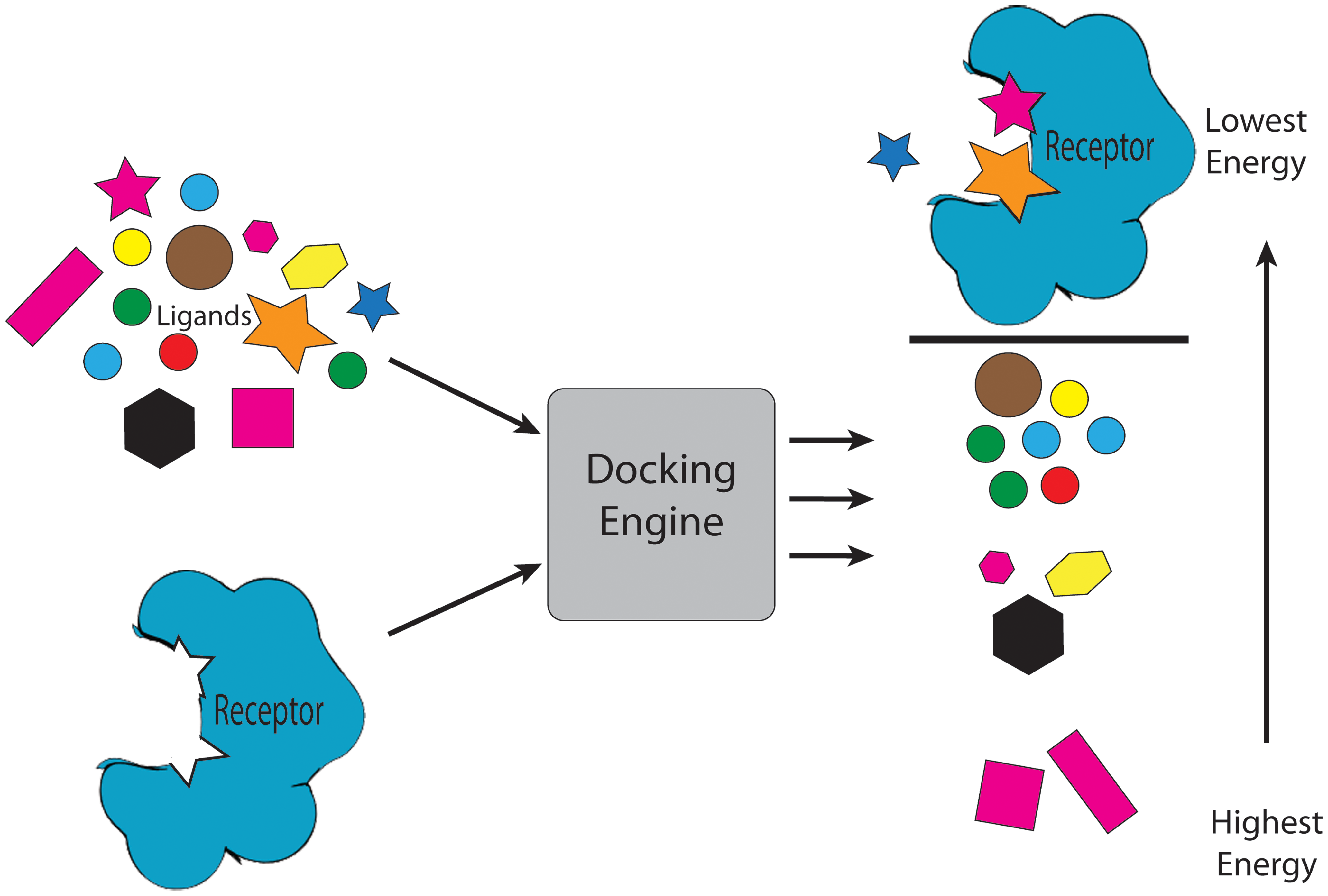
Ranking of molecules using molecular docking. Taken from Jacob et al.
In the early 20th century, Paul Ehrlich laid the intelectual foundation of modern drug-design research through his inspirational concept of ‘magical bullets’. These ‘bullets’ are small chemical compounds with the ability to bind to biological targets, such as proteins or nucleic acids, with ‘magical’ specificity. This interaction affects the structure and dynamical properties of the target, and therefore alters their function. Fast-forward 100 years and the current paradigm is still the development of specially tailored drugs to target proteins (or other bio macromolecules) which might be crucially involved in the molecular mechanism of a given disease.
Nowadays, we can use simulations to understand the binding process of a drug to its target with an atomic level of detail through computation of their energy of interaction. Not only that, but the potency of existing drugs can be improved, or new ones can be designed from scratch. One of the most widely applied techniques in computer-aided drug-design (CADD for the acronym lovers) is molecular docking, which can quickly calculate the energy of a drug-protein interaction. Using this approach, we can perform ‘virtual screening’ early in the drug-discovery process: a library of ligands (usually tens of thousands) is ranked based on their predicted affinity for a certain target. A lot of time and money can be saved if only the compounds with a good predicted affinity are further studied experimentally using in vitro activity assays.
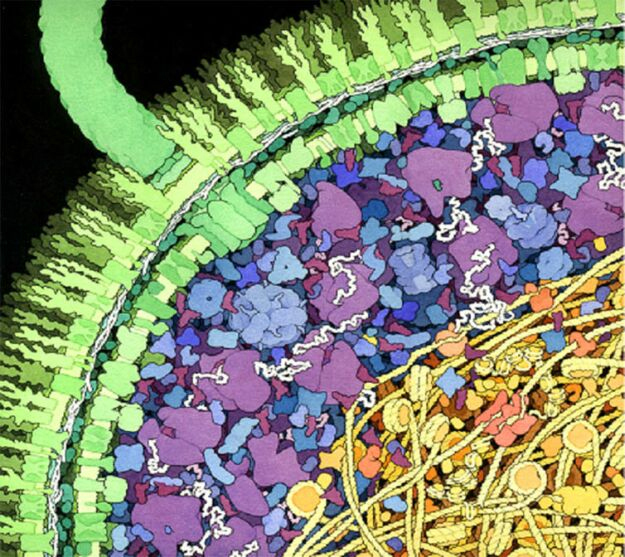
Artistic rendering of the inside of a cell. There is a high degree of compartimentalization, and macromolecules can take up to 30% of the total volume. Taken from Hoppert and Mayer.
A particularly hard challenge for drug discovery are the effects of polypharmacology. Inside the cell, drugs interact with multiple targets, possibly leading to side-effects and toxicity issues. This is mainly caused by two phenomena: macromolecular crowding inside the cell, and the coexistence of protein families which share structural similarities. The cell is a tightly packed environment, which deviates from the setup that is used in virtual screening calculations – the targets are never in isolation, but rather interacting with many other molecules. This can have important implications in the process of ligand binding, but at the moment this bewildering complexity is impossible to take into account at the computational stage of drug-development.
The second issue is more easily tractable through molecular docking, and it’s what my project is aimed at. If we want to target a protein inside a family with specificity, all we need to do is dock the same library of ligands on all the other available structures in the protein family. If there are multiple crystal structures for the same protein (proteins are not static objects, and therefore they can be crystallized in different conformations), we should use them as well. The aim is then to select the compounds that score best for the different structures of our target, and worse for the other members of the family, ensuring then an acceptable level of specificity. This would mean that only the most magical bullets of them all would be further studied experimentally. In the next post I will give more details on how I plan to implement this re-ranking algorithm in Python as a backend of the currently available ChemBioServer.
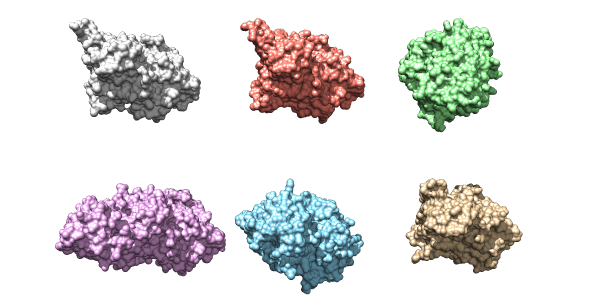
The six kinase structures that will be used as a data set for the project. Their structural similarities are evident.