Farewell…
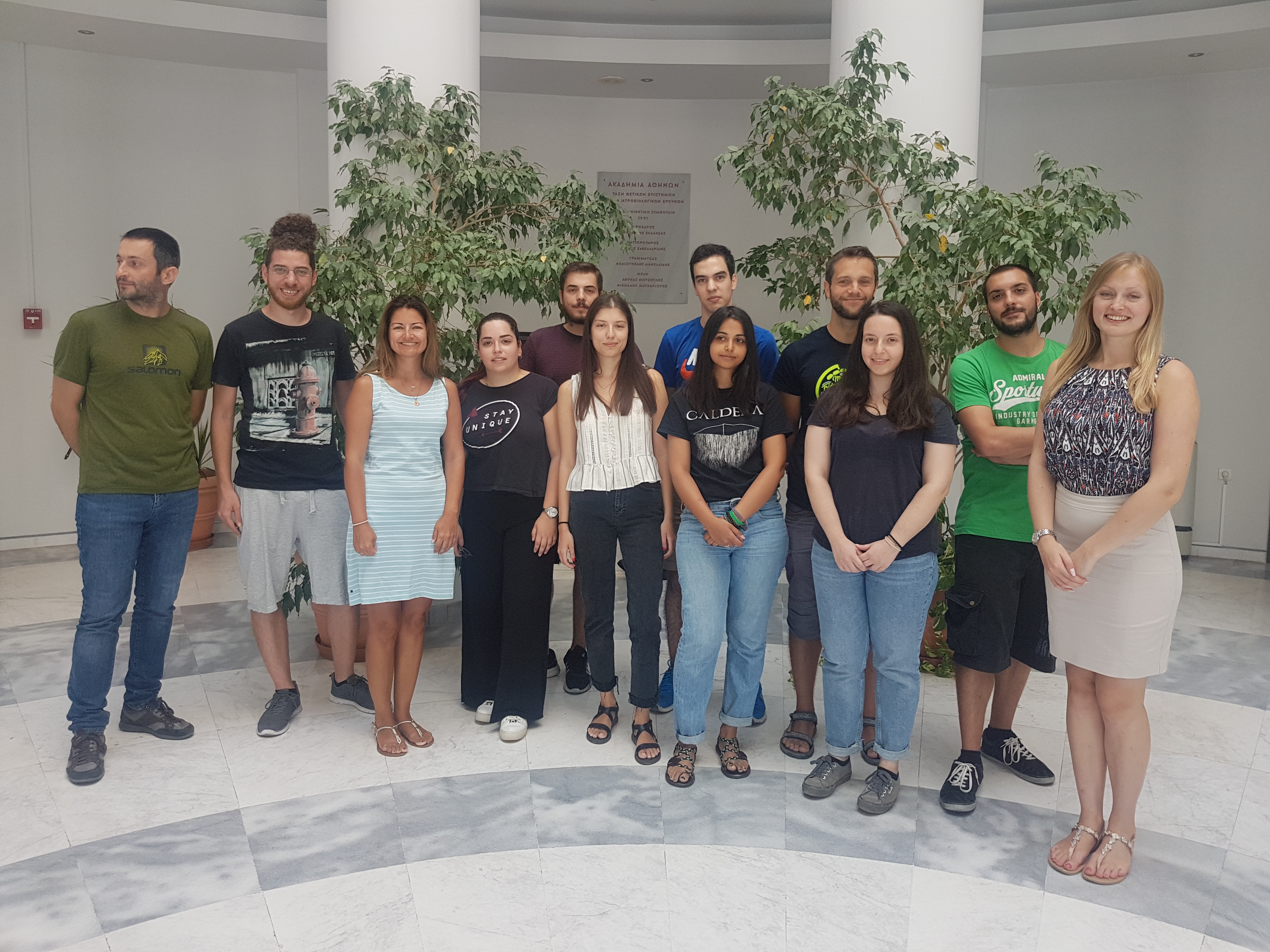
In the German movie Good bye, Lenin, a proud socialist woman falls into a comma in October 1989. When she wakes up again, the Wall has already fallen. To protect her health, her son decides to hide this historical episode from her. She would suffer a huge anguish if she knew her loved old regime has disappeared. Then, the son builds a parallel reality inside their apartment, where nothing has changed, a bastion of the old German Democratic Republic. How is this related to my Summer of HPC project? We’ll see…
Project recap
As we have learnt in the previous blog posts, drug discovery is a very expensive (~US $2.8 billion) and slow (12 to 15 years) process. Free Energy Perturbation (FEP) calculations coupled with Molecular Dynamics simulations may allow us to speed it up and reduce the cost and time of efficacy optimization (one of the goals of Lead Optimization, which in turn is the most expensive pre-clinical phase of drug discovery). These simulations allow, in theory, to computationally screen many compounds, select those with better binding affinity of their target and synthesize only the most promising ones, thus saving valuable resources and time. The goals of this project have been 1) to compare FEP results with experimental data to prove whether it is accurate enough to be used in industry, and 2) whether High-Performance Computing (HPC) is necessary for FEP/MD calculations. All simulations were performed on ARIS supercomputer, the national Greek supercomputer.
Project Results
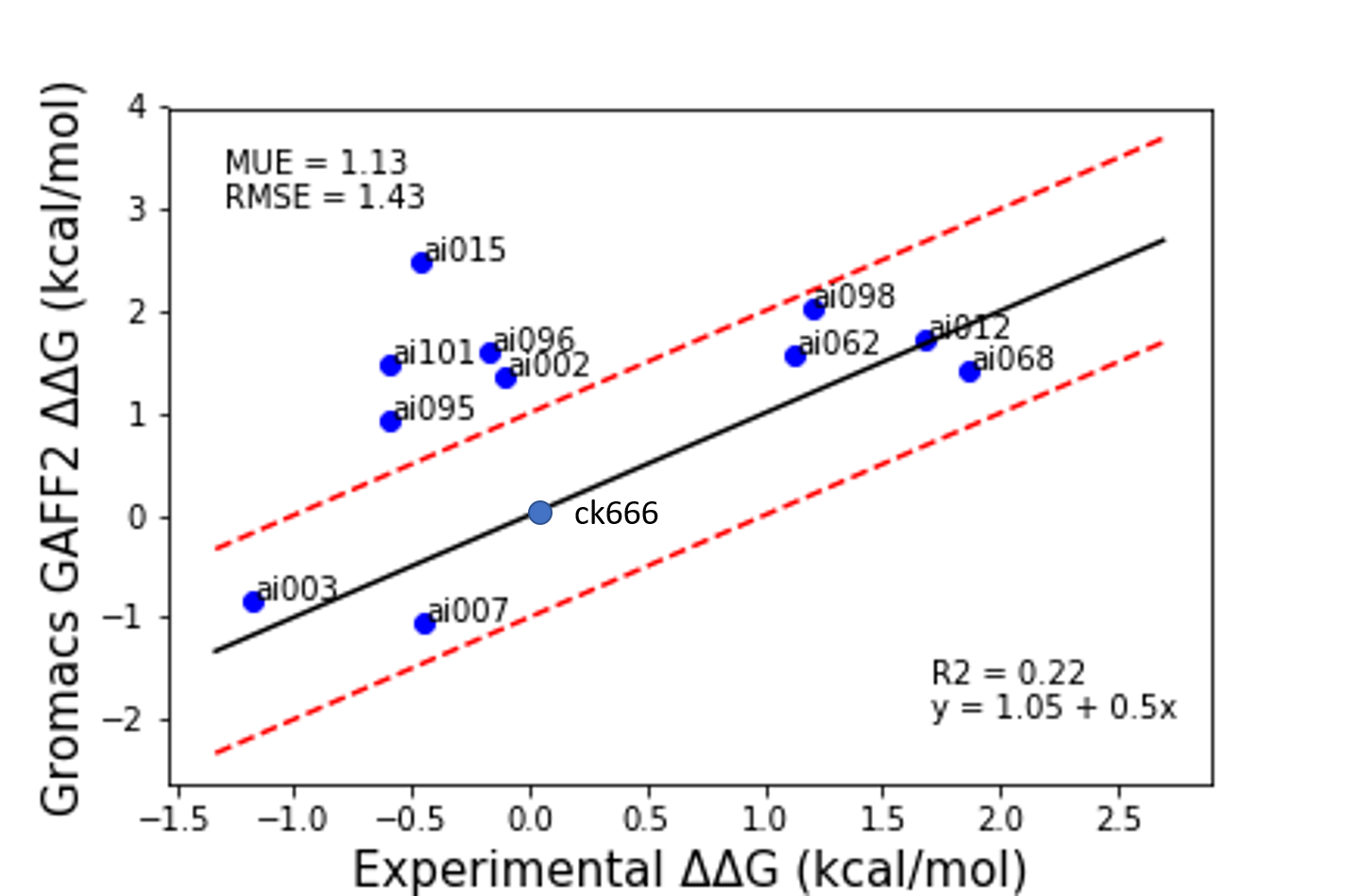
Our test case has been CK-666. It is a micromolar inhibitor of protein Arp23. This protein is involved cell movement and in tumor cells migration. However, when CK-666 binds to it, the protein is inactivated. Different modifications of CK-666 (analogs), were studied.
After completing FEP/MD simulations for 11 analogs against CK-666 we could generate a correlation plot with the experimental results. How did our predictions correlated with experiments? That’s the big question.
When looking at the final correlation plots, it is observed how, for example, FEP simulations using Gromacs software and the GAFF2 force field correctly predicted that molecules ai003 and ai007 are favored over the reference ligand (CK666), because their relative free energy is smaller than zero. For other analogs, such as ai015 or ai101, this was not the case. But, the Mean Absolute Error was 1.13 kcal/mol, which allows us to say that FEP can predict whether an analog will be better or worse than the originally synthesized molecule with a ΔΔG of ~1 kcal/mol. Thus, this technique has the potential to greatly reduce the cost and time of lead optimization for a drug to enter clinical trials.
Finally, we showed that HPC resources are essential for FEP/MD. On average, 13.5 hours and 1680 cores were needed for the complex phase of FEP, while 6.5 hours and 210 cores were necessary for the solvent phase (see here the hardware specifications). And that is for one single compound. Since the goal of FEP is to screen many analogs to select only a few of them to be synthesized, let’s imagine the scenario in which we want to analyze 100 analogs in 1 day. This would require 5250 cores for the solvent phase of the simulations, and 84000 cores for the complex phase.
One of the last stages of the project included creating a popular video in which the entire work performed is explained, together with the results and conclusions. If you are eager to learn more at what I have done, please, check it here!
Farewell
Hopefully, the day when drug discovery cost is reduced thanks to the integration of computational tools in the pipeline will arrive in the near future. However, there is still work to be done by industry and academia in this direction. Meanwhile, from next week on, the only good times I will be missing will be my two months at Athens. Perhaps I will ask my family to pretend I am still there…
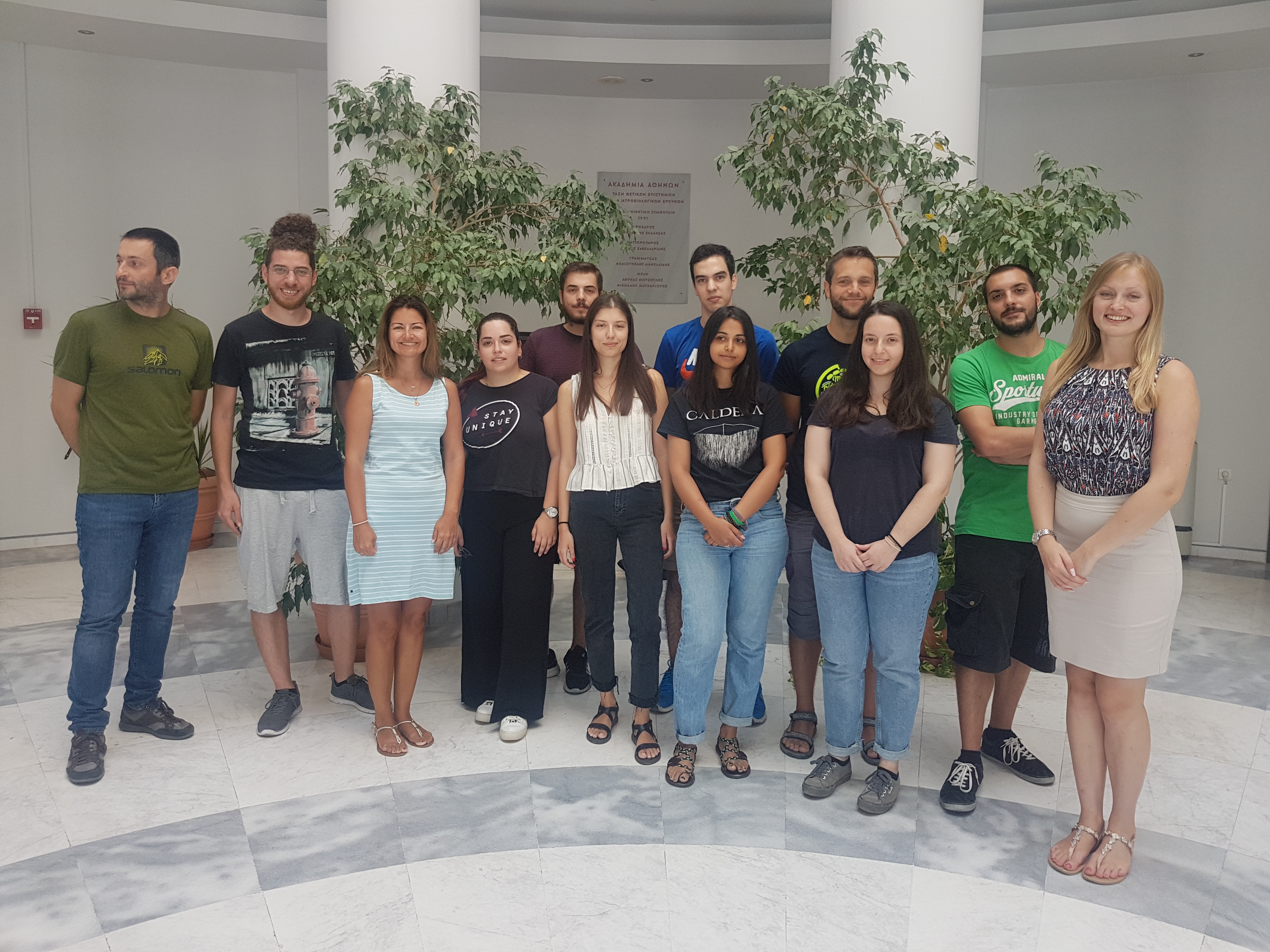