Project reference: 2011
Simulations of classical or quantum field theories often rely on a lattice discretized version of the underlying theory. For example, simulations of Lattice Quantum Chromodynamics (QCD, the theory of quarks and gluons) are used to study properties of strongly interacting matter and can, e.g., be used to calculate properties of the quark-gluon plasma, a phase of matter that existed a few milliseconds after the Big Bang (at temperatures larger than a trillion degrees Celsius). Such simulations take up a large fraction of the available supercomputing resources worldwide.
Other theories have a lattice structure already “build in”, as is the case for graphene, with its famous honeycomb structure. Simulations studying this material can build on the experience gathered in Lattice QCD. These simulations require, e.g., the repeated computation of solutions of extremely sparse linear systems and update their degrees of freedom using symplectic integrators.
Depending on personal preference, the student can decide to work on graphene or on Lattice QCD. He/she will be involved in tuning and scaling the most critical parts of a specific method, or attempt to optimize for a specific architecture in the algorithm space.
In the former case, the student can select among different target architectures, ranging from Intel XeonPhi (KNL), Intel Xeon (Haswell/Skylake) or GPUs (OpenPOWER), which are available in different installations at the institute. To that end, he/she will benchmark the method and identify the relevant kernels. He/she will analyse the performance of the kernels, identify performance bottlenecks, and develop strategies to solve these – if possible taking similarities between the target architectures (such as SIMD vectors) into account. He/she will optimize the kernels and document the steps taken in the optimization as well as the performance results achieved.
In the latter case, the student will, after getting familiar with the architectures, explore different methods by either implementing them or using those that have already been implemented. He/she will explore how the algorithmic properties match the hardware capabilities. He/she will test the archived total performance, and study bottlenecks e.g. using profiling tools. He/she will then test the method at different scales and document the findings.
In any case, the student is embedded in an extended infrastructure of hardware, computing, and benchmarking experts at the institute.
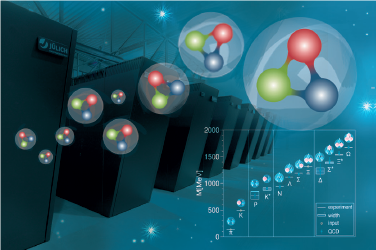
QCD & HPC
Project Mentor: Dr. Stefan Krieg
Project Co-mentor: Dr. Eric Gregory
Site Co-ordinator: Ivo Kabadshow
Participants: Anssi Tapani Manninen, Aitor López Sánchez
Learning Outcomes:
The student will familiarize himself with important new HPC architectures, such as Intel Xeon, OpenPOWER or other accelerated architectures. He/she will learn how the hardware functions on a low level and use this knowledge to devise optimal software and algorithms. He/she will use state-of-the art benchmarking tools to achieve optimal performance.
Student Prerequisites (compulsory):
- Programming experience in C/C++
Student Prerequisites (desirable):
- Knowledge of computer architectures
- Basic knowledge on numerical methods
- Basic knowledge on benchmarking
- Computer science, mathematics, or physics background
Training Materials:
Supercomputers @ JSC
http://www.fz-juelich.de/ias/jsc/EN/Expertise/Supercomputers/supercomputers_node.html
Architectures
https://developer.nvidia.com/cuda-zone
http://www.openacc.org/content/education
Paper on MG with introduction to LQCD from the mathematician’s point of view: http://arxiv.org/abs/1303.1377
Introductory text for LQCD:
http://arxiv.org/abs/hep-lat/0012005
http://arxiv.org/abs/hep-ph/0205181
Introduction to simulations of graphene:
https://arxiv.org/abs/1403.3620
https://arxiv.org/abs/1511.04918
Workplan:
Week – Work package
- Training and introduction
- Introduction to architectures
- Introductory problems
- Introduction to methods
- Optimization and benchmarking, documentation
- Optimization and benchmarking, documentation
- Optimization and benchmarking, documentation
Generation of final performance results. Preparation of plots/figures. Submission of results.
Final Product Description
The end product will be a student educated in the basics of HPC, optimized methods/algortithms or HPC software.
Adapting the Project: Increasing the Difficulty:
The student can choose to work on a more complicated algorithm or aim to optimize a kernel using more low level (“down to the metal”) techniques.
Adapting the Project: Decreasing the Difficulty:
Should a student that finds the task of optimizing a complex kernel too challenging, could restrict himself to simple or toy kernels, in order to have a learning experience. Alternatively, if the student finds a particular method too complex for the time available, a less involved algorithm can be selected.
Resources:
The student will have his own desk in an open-plan office (12 desks in total) or in a separate office (2-3 desks in total), will get access (and computation time) on the required HPC hardware for the project and have his own workplace with fully equipped workstation for the time of the program. A range of performance and benchmarking tools are available on site and can be used within the project. No further resources are required.
Organisation:
Jülich Supercomputing Centre
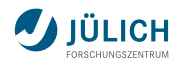